Our Services
Virtual HTS: Hit Identification
Our AI models learn from your data and can be used to virtually screen any libraries of your choice or all publicly available libraries, up to billions of compounds. You get a qualified hit list annotated with ADMET and other selected relevant properties, such as fluorescence, specific toxicity, etc.
Data confidentiality — Since we just need your activity scores and the molecules’ structures, we offer the options to train from your datasets without knowing your target or property of interest. Alternatively, if you have no or little data, or if you cannot disclose it, we can train from our data only, provided that the target is disclosed and available in our database. In any case, your data will be securely stored and kept strictly confidential.
Virtual HTS: Hit Identification
We provide pharmacological property predictions for any small molecule up to ~3000 Daltons. Our ADMET (Absorption, Distribution, Metabolism, Toxicity) encompass >20 properties including:
- Permeability
- Solubility
- Cytochrome inhibition
- Toxicity
Off-Target Prediction: Lead Optimization
Our curated database contains a large number and variety of targets, giving us the ability to predict off-target activities of compounds for effective hit prioritization.
De novo predictions: Lead Optimization
Our system can screen virtual new compounds or analogs to existing hit compounds for hit-to-lead and lead optimization. This enables the virtual selection of many more hit analogs prior to costly syntheses and experiments, which drastically accelerates the hit-to-lead and lead optimization process.
Global discovery and preclinical service
One of the unique features of our system is the fact it is designed from the grounds up for multi-task learning and prediction, obtaining significantly better understanding of specific chemistry of each compound with each additional data point. This allows us to combine different measurements on the same libraries and obtain prediction accuracies beyond experimental. Multiparametric predictions enable us to parallelize hit discovery with lead optimization, leading to considerable gains in effectiveness and speed.
Our AI model led to the discovery of novel antivirals against COVID-19.
We trained our neural networks on various experiments encompassing SARS-CoV-2 inhibition and cellular toxicity. Then we used the AI’s predictions to select 6 compounds among all drugs that have been validated for human trial and never investigated for their activity against COVID-19.The predictions of new anti-SARS-CoV-2 drug candidates were confirmed in vitro with an exceptional success rate in multiple cellular assays.
The experiments were made within a collaboration with a virology lab in Denmark.
- 5 of these drugs displayed antiviral activity in SARS-CoV-2 infected cells as predicted, at non-toxic doses
- Only one of these drugs is a known antiviral
SARS-CoV-2 Predictions Validated in Cell Assay
Experimental activity of our predicted SARS-CoV-2 inhibitors in cell cultures
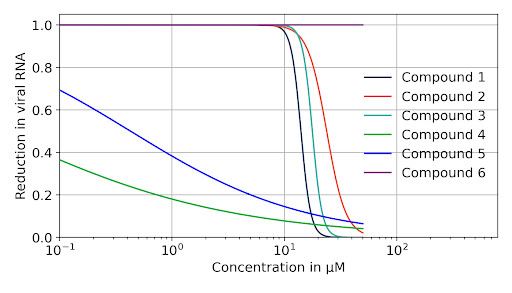
After our AI model’s predictions led to the discovery of new antivirals against SARS-CoV2 in partnership with the Danish lab, we have performed another in vitro validation with a laboratory in California using a different method (Luciferase-based cellular assay) and molecular library. This collaboration identified two more active compounds.
AI-powered platform finds compounds killing cancer cells.
We were provided with a training set on cancer cell viability from high-content imaging data. Our predictions were tested on withheld compounds (unpublished data hidden from us). A cancer research institution in Australia provided us with the data and conducted this proof of concept.
The results showed that our predictions were as precise as experimental reproducibility to determine compounds potentially killing cancer cells.
viability threshold
|
reproducibility AUC* (%)
|
predictions AUC* (%)
|
0.3
|
87.3
|
±1.8
|
85.1
|
±0.8
|
0.5
|
78.0
|
±1.9
|
81.3
|
±0.8
|
Cancer Cell Viability Prediction Validation with High-Content Imaging
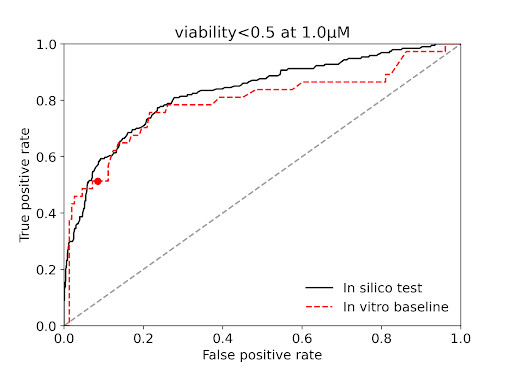
*AUC: a standard robust measure of predictions accuracy (probability that an active compound is scored higher than an inactive one).
This shows the versatility and accuracy of our therapeutic field agnostic technology.
Discovery of new preclinical candidates against HIV-1.
Our AI model was trained on a PubChem assay screening for GP41 (HIV-1) inhibitors. We commissioned a Norwegian HTS partner to proceed to an in vitro screen of GP41 inhibition in a molecular assay. We picked the available small library (1950 new compounds) with the most predicted hits. In order to validate the potential of our technology within screening contexts, we committed our ranked top 10% predictions prior to the experiment for independent confirmation by our partner.
22 hits were found in the library, which corresponded to our predictions.
- 2 hits were found in our top 3 predictions.
- 20/22 hits were in our top 10% predictions, showing the enrichment capabilities of our technology.
The probability of this happening by chance (p-value from random predictions) is 1 in 1018
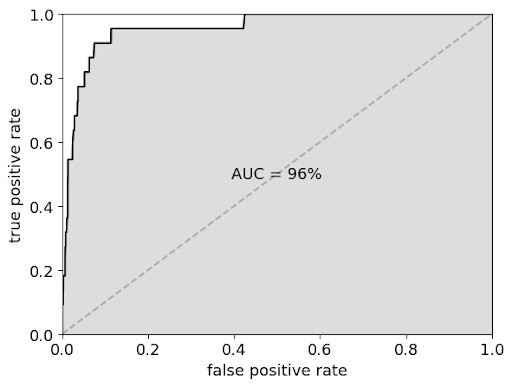